AI’s Energy Footprint Warrants Markets, Not Panic
Escalating electric power demand from artificial intelligence (AI) has prompted a range of media, political, and regulatory responses including congressional hearings, state policy actions, and increased focus in energy forums. Experts and policymakers have been especially concerned that power-hungry AI may erode grid reliability, raise energy costs for everyone, and skyrocket emissions. Further concerns, including those of presidential candidates, have surfaced that the inability to meet AI energy demand domestically poses strategic risks, as data centers might relocate offshore.
This makes a snapshot of policy implications imperative. While initial analyses are in, the true extent of AI’s impact on energy systems remains highly uncertain. Analyses are often outdated within months of publication, with rapid revisions evident in demand-forecasting assumptions. Forecasts themselves are often questioned due to inherent regulatory incentives to inflate them. But enough is known to begin to profile AI’s energy and environmental footprint and frame a productive legislative and regulatory response. In short, while AI’s energy profile does not seem to present major economic or environmental concerns in a healthy marketplace, it amplifies the preexisting case to fix flawed public policy.
Industry Background
To understand the effects of AI on electricity costs and reliability, it is important to understand how new demand affects electric infrastructure and operations. Generally, electric infrastructure is sized to meet peak demand and operates with large reserves the vast majority of the time. For example, half of congestion costs on transmission lines came during only 5 percent of hours in recent years. Similarly, ample generation is available most any time, which is why generation adequacy concerns are commonly assessed for extreme weather in the top 10th percentile. Put another way, on most days, less than half of available infrastructure and capacity is needed to meet demand. This is why the shape of new demand—especially whether it is flexible on-peak—carries more infrastructure implications than its overall consumption volume.
The location of new demand also carries major infrastructure implications. Transmission and distribution systems have flow limitations, while generation is becoming more geographically dependent. All this results in high spatial variance in the cost to serve new demand. In fact, limitations on the transmission system to export power from generation-rich areas (such as windy areas) increasingly require grid operators to curtail power production even if doing so would improve reliability and lower cost elsewhere on the system. Consequently, there is slack on the power system the vast majority of the time, especially in certain locations. At the same time, certain locations face persistently tight supply-demand conditions because of chronically saturated infrastructure.
As such, the size, shape, and location of AI’s energy profile has major bearings on its electric cost and reliability implications. It turns out that the same characteristics profoundly affect its emissions profile as well. That is, the emissions of marginal power demand fluctuates by multiples depending on location and real-time supply-demand balance on the grid. In short, the more flexible AI is in operating and siting new data centers, the lower its environmental footprint and strain on electric infrastructure.
The regulatory paradigm influences the location and flexibility of power demand as well as the speed, cost, emissions, and risk profile of new supply infrastructure. States with competitive wholesale and retail electric markets harness competitive forces to drive generation decisions. Markets provide granular temporal and spatial price signals to incent demand to locate and operate flexibly. States with traditional cost-of-service regulation lack these incentives because the monopoly utility’s financial motive is to expand the asset base upon which it earns a regulated return.
Transmission and distribution (T&D) infrastructure is mostly on cost-of-service regulation. T&D regulatory architecture—including variances in planning transparency, use of cost-benefit analysis, competitive bidding, and other features that affect the economic performance of T&D expansion—varies by region and state. All categories of power infrastructure face growing permitting and siting challenges, which are especially problematic in an era of resurgent load growth.
The mesh point of regulatory architecture with AI’s energy profile shapes the cost, reliability, and environmental implications of AI energy needs. A peer lesson comes from cryptocurrency, which is extremely flexible in its siting and operational profile. Crypto miners increasingly locate in renewables-rich areas of states with competitive electricity markets, consume when supplies are ample, and curtail their power consumption when grid conditions tighten. Thus, despite a boom in power consumption, crypto mining has resulted in low incremental infrastructure and emissions impact. This would not be the case with inflexible demand growth in monopoly utility footprints.
AI’s Energy Profile
Figure 1
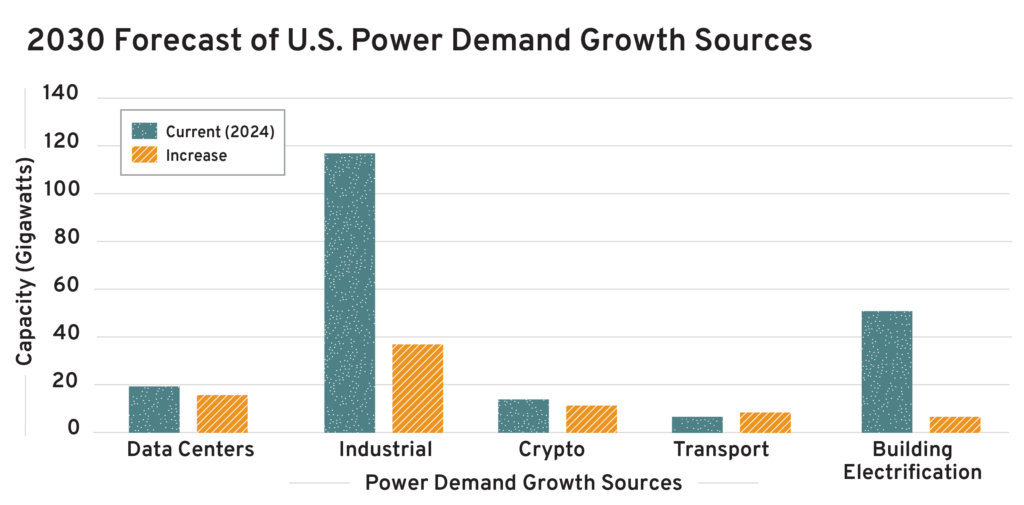
Source: Chart created from R Street analysis of Brattle Group data
A series of reports and conversations shed light on AI’s emerging energy profile. Key profile characteristics include demand size, location, shape, and reliability and environmental preferences.
Observed trends and expected conditions leave us with six takeaways from AI’s energy profile:
- AI is a formidable but minority driver of electric demand growth expectations. To date, data center demand is about 19 gigawatts (GW). For context, one GW is the size of a conventional nuclear reactor, and the United States has about 1,200 GW of generation capacity. One prominent forecast projects data center demand to grow 16 GW by 2030, constituting 20 percent of total projected growth (see Figure 1). This is a 9 percent compound annual growth rate for data center demand. Other reports expect higher growth, at 50 GW of data center capacity expansion by 2028. Another prominent industry group predicts that data centers could account for 5 to 9 percent of domestic electricity consumption by 2030. There is also evidence of potentially inflated forecasts resulting from duplicative measurement error. This happens because although data centers request capacity in multiple utility footprints to expand a single data center, each request is built into utilities’ independent forecasts. Altogether, while data centers currently constitute a formidable minority share of load growth, they may comprise a modest-to-moderate share of total U.S. power demand by the next decade. Importantly, AI is only a subset of data center power use; however, forecasts typically do not distinguish AI from non-AI uses. Specifically, AI demands higher power density, increased storage capacity, and greater computing power within data centers.
- The timing of AI demand growth is sudden. Load growth expectations have been revised upward dramatically over the last two years, due in part to AI. Beginning in 2022 (and especially in 2023), a surge in data center and industrial development caused sudden, large increases in five-year load growth expectations (increase of 18 GW in the 2028 summer peak demand forecast). The power industry often struggles with sudden shifts because of infrastructure’s three-to-10-year financing and construction lead times, with the regulatory climate adding five to 10 years for most projects. This supply lag means that resurgent load growth may result in supply-demand disequilibrium for an extended period, with effects highly dependent on where load growth occurs.
- AI apparently has more siting flexibility than traditional data centers. Data centers are highly concentrated demand sources, sometimes referred to as “point loads.” Traditional data centers were built out in geographic clusters, prioritizing non-energy siting criteria like network connectivity. This led to concentrated power infrastructure challenges, such as upgrading the local and regional transmission system to accommodate Data Center Alley in Virginia. Encouragingly, recent data center development exhibits considerable siting flexibility. Notably, data centers are seeking to expand nearby or at the site of existing carbon-free power plants, thereby reducing infrastructure strain and emissions compared to locating elsewhere. Such power system siting factors for data centers has coincided with the rise of AI and perhaps in response to tightening power infrastructure conditions. Siting location may be more flexible as customer latency becomes less of a constraining factor, especially with AI training. However, because low-latency connectivity remains key to enterprise cloud customers, data center proximity needs will drive continued growth in constrained areas like northern Virginia. With various areas reaching power infrastructure saturation while others maintain considerable slack, the responsiveness of data center siting to power system constraints is critical to monitor.
- AI’s demand shape is not well known, but it is possibly more flexible than conventional data centers. Conventional data centers have a flat, baseload demand shape with virtually no operational flexibility. Such rigidity is challenging for power systems to accommodate during system scarcity events. AI power demand has the potential to be less flat and more flexible, depending on major unknowns like the proportion of AI used for training versus inference purposes. Some reports suggest the potential to batch AI queries, which could result in more flexible power consumption. There are indications that AI will be more operationally flexible than conventional centers and less flexible than crypto mining. Such a wide range provides little clarity, but even a semblance of potential operational flexibility is noteworthy.
- AI may have a high willingness to pay for reliable service. Conventional data centers exhibit a high value of lost load (the economic value of avoiding an outage) relative to other power uses. It is unclear how different AI applications compare in terms of their sensitivity to continuous supply. If AI applications have similarly high reliability requirements, they might result in less-flexible power demand. If they do, then it will also increase the value of on-site power. Data centers have explored various forms of on-site power as primary and backup sources, which can greatly increase the net demand flexibility of power draws from the central grid. In open electricity markets, large consumers with ample on-site power sometimes become net exporters of power to the central grid during grid emergencies.
- Data center developers exhibit a substantial willingness to pay for clean energy. Data center developers have ambitious decarbonization goals, employ internal prices on carbon, and factor proximity to low emissions energy resources into their siting decisions. AI has contributed to energy demand that was unexpected when tech firms set their initial environmental targets. This has caused tech firms to fall behind on their targets; however, they remain committed, recalibrating their targets and action plans based on new expectations. This indicates that despite regression on absolute emissions reductions, the willingness to pay for lower emissions on a per-unit energy consumption basis has remained. Progress will not be linear.
- AI’s energy and emissions profile is highly uncertain. Four sources of uncertainty are key: the economic demand for AI (workload), AI energy efficiency improvements, AI siting and operational flexibility, and tech firms’ willingness to pay to decarbonize. The level of AI power demand growth by 2040 varies by roughly an order of magnitude across numerous projections and scenarios. The workload alone is immensely hard to predict, with uncertain factors including the pace and extent of corporate AI adoption. Furthermore, energy efficiency improvements are rapidly evolving across data center demand components—especially computing and cooling. Plausible scenarios in AI energy efficiency may reduce most power consumption that would otherwise occur by 2030. AI energy demand flexibility is largely unknown, while uncertainty in data center siting behavior is equally important. For one thing, data centers require less energy for cooling purposes in colder climates, such as in North Dakota. The degree of data centers’ long-run sensitivity to power costs and emissions impact is unclear, but potentially transformative. Lower power costs, infrastructure strain, and emissions from power consumption are correlated and vary widely across the country. If these become major data center siting criteria, AI’s energy and emissions effects may plummet.
Policy Implications
AI’s energy profile underscores the value of free and competitive markets. States with competitive electricity markets and low permitting barriers should outperform on economic, reliability, and environmental grounds. Aside from Texas, however, nearly all states have unnecessary monopoly utilities and/or restrictive permitting practices. Federal permitting reforms have momentum, but state permitting restrictions have grown sharply throughout the past decade, threatening the retention of economical existing infrastructure and the expansion of new facilities. New power plants in most regions face five-year wait times just to receive approval to interconnect to the regional grid.
To a degree, competitive electricity markets are incenting new demand, including AI, to locate where there is infrastructure slack, to operate more flexibly, and to adopt on-site generation. Most prominently, tech firms are collaborating with competitive power plant owners to build out data centers at the site of nuclear plants. This development, known as “co-location,” is a welcome sign of market creativity. In addition to nuclear co-location, high wind-producing areas routinely have surpluses of local, emissions-free generation that attracts data center development. Such practices reduce infrastructure burdens and lower per-unit emissions from data center expansion. This explains why large consumers, including data centers, seek reforms to give consumers direct market access in these areas.
By contrast, monopoly utilities have a track record of suppressing demand flexibility, on-site generation, and incentives to locate demand where it is least burdensome to infrastructure. It is little surprise, then, that monopolies are slow to adapt to AI’s energy needs, even denying service unless data centers pay new rates. Distribution and local transmission investments are made exclusively by monopoly utilities out of necessity, but grid upgrades for new data centers are overly slow and expensive given utility system opacity and the lack of least-cost investment analyses.
Regression is a major concern in competitive states like Ohio and Pennsylvania, where distribution-service monopolies are vying for states to re-monopolize generation despite consumer opposition. This would force captive ratepayers to finance monopoly generation to meet new demand. Doing so would displace lower-cost generation from competitive suppliers and exacerbate system-wide investment risk. Such cross-subsidies fundamentally compromise the integrity of competitive markets.
Distortive market interventions are often motivated by the perceived reliability risk that is amplified by demand growth. Yet markets are especially advantageous under high certainty, which is perhaps the single best characterization of AI energy demand. Competitive suppliers absorb risk and manage it well, whereas monopoly utilities socialize risk and have a track record of overbuilding supply and choosing overly expensive supply options. Markets also outperform when consumers have diverse preferences, and AI developers have unique preferences for service reliability and emissions avoidance.
States with competitive electricity markets and consumer choice are easier for green-minded consumers—including AI developers—to contract for clean energy. This explains why Texas has become the country’s clean energy leader. Regions like the Mid-Atlantic that publish locational marginal emissions data make it possible to account for the indirect emissions from power consumption. Adopting this in Texas, for example, could double the carbon displacement of clean energy deployment. Despite green appetite from power consumers including AI, unclear and overreaching financial regulation has deterred suppliers from developing innovative products that best reduce the indirect emissions of power consumption.
Several policy takeaways from AI’s energy growth:
- Prepare for load growth generally, not disproportionately for AI. Most load growth is coming from demand besides AI; thus, the policy implications of load growth in totality should take precedence. Load growth remained flat for almost the entirety of this century, which helped mask imperfections in permitting and electricity policy. Resurgent load growth makes these problems more pressing to remedy.
- Streamline permitting, finish generator interconnection reforms, improve electricity competition, and enhance T&D policy. Policymakers should expand and improve electricity markets and consumer choice, which hold a clear cost, risk, and reliability edge for handling load growth. Just as important is avoiding regression, such as not letting monopoly utilities contaminate markets by forcing ratepayers to subsidize new generation. Policymakers should also prioritize responsible permitting and generator interconnection reform to reduce litigation risk and shave years off infrastructure development timelines. A variety of economical T&D reforms could alleviate immediate load-growth challenges, such as optimizing the existing system to slash transmission congestion, as well as longer-term reforms like proactive and transparent planning, use of robust cost-benefit tests, and competitive bidding (where appropriate) to boost T&D capacity far more economically than the status quo.
- Deepen understanding of AI energy demand, especially improved forecasting. Researchers, regulators, and policymakers should expend appropriate resources to close the large knowledge gap on AI’s energy footprint. This includes not only its volume, but also—and more importantly—its load shape, general location, and specific co-location potential. Determining actual infrastructure impacts will require better information and modeling. AI’s demand profile and infrastructure impact is a function of regulatory architectures, which forecasters have historically not accounted for. Additionally, efforts must be made to avoid double-counting of AI-related energy developments so as to ensure accurate assessment of their cumulative impact. Better understanding the relationship between AI growth relative to energy efficiency gains is essential, along with associated metrics (e.g., energy per unit of computing). In the meantime, policymaking must continue with the acknowledgement of a high level of AI load growth uncertainty.
- Expand information and reduce barriers to voluntary AI environmental improvement. AI developers are environmentally motivated, such that policy ideas to force or subsidize clean practices are ill advised. Rather, policymakers should enable voluntary clean practices. One priority is the provision of environmental information, such as the publication of location-based marginal emissions. Another is to clarify light-touch financial regulation around greenwashing and voluntary carbon markets to unlock chilled capital, avoiding an ambiguous, heavy-handed approach that would likely spook innovators and induce corporate liabilities.